All About : Neuromorphic Computing
Neuromorphic Computing: Revolutionizing the Future of Brain-Inspired Technology
Introduction
In a world where traditional computing is reaching its limits, the search for more efficient, adaptive, and intelligent systems has given rise to a new frontier: neuromorphic computing. Inspired by the structure and processes of the human brain, neuromorphic computing is a technological marvel that holds the potential to transform industries ranging from healthcare to artificial intelligence (AI).
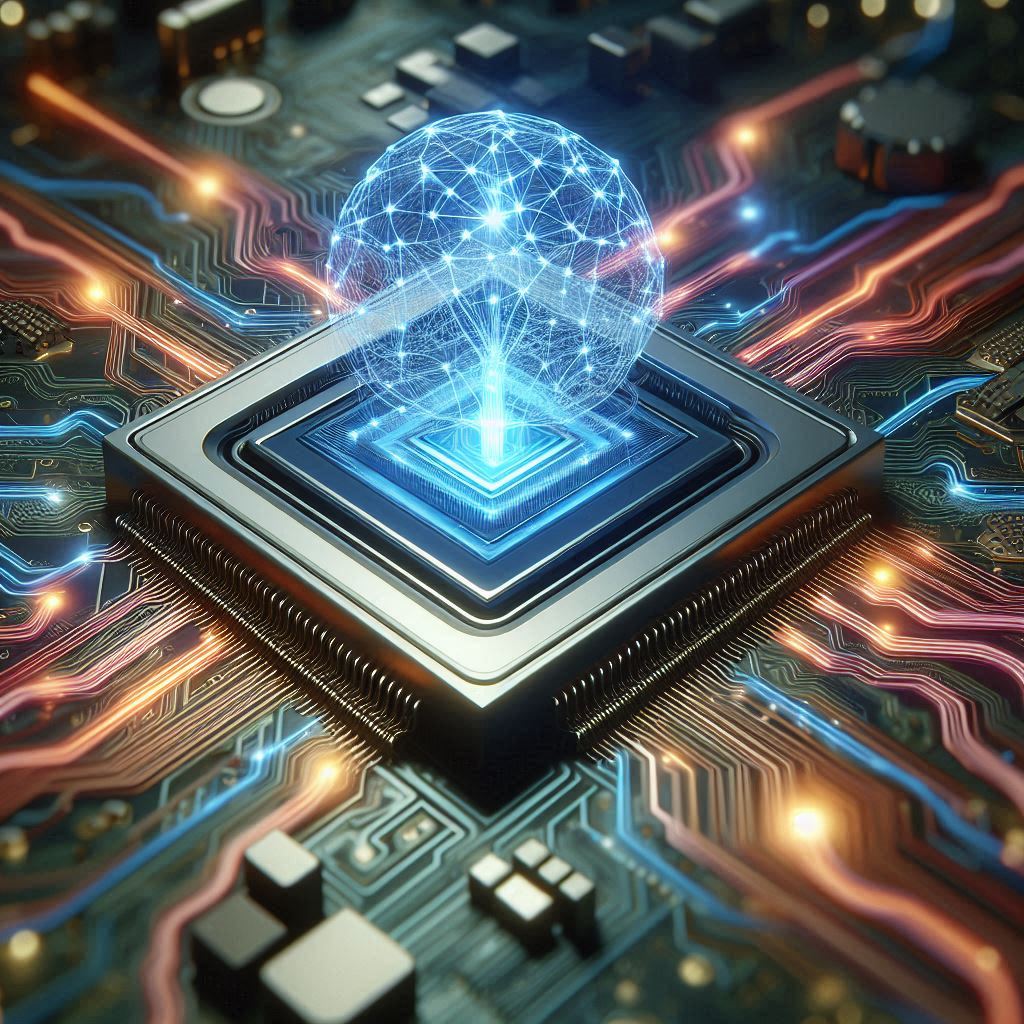
In this blog, we’ll explore the fundamentals of neuromorphic-computing, how it works, its unique advantages, and its groundbreaking applications. We’ll also examine the challenges and future outlook for this rapidly evolving field.
What Is Neuromorphic Computing?
Neuromorphic-computing refers to the design and development of computer systems that mimic the neural and synaptic architecture of the human brain. Unlike traditional computing, which relies on binary processing and pre-defined algorithms, neuromorphic systems aim to replicate the brain’s ability to learn, adapt, and process information in parallel.
Key Features:
- Brain-Inspired Design: Neuromorphic hardware and software are modeled after neurons and synapses, enabling more natural information processing.
- Event-Driven Processing: These systems operate only when triggered by specific events, significantly reducing energy consumption.
- Parallelism: Neuromorphic computing can handle multiple tasks simultaneously, making it highly efficient for complex computations.
The term “neuromorphic” was first coined by Carver Mead in the 1980s, and the field has since evolved with advancements in neuroscience, materials science, and artificial intelligence.
How Does Neuromorphic Computing Work?
Neuromorphic-computing systems use spiking neural networks (SNNs) to simulate the way biological neurons communicate through electrical impulses or “spikes.” Unlike artificial neural networks (ANNs) in traditional AI, which process information in a linear manner, SNNs mimic the dynamic nature of the brain.
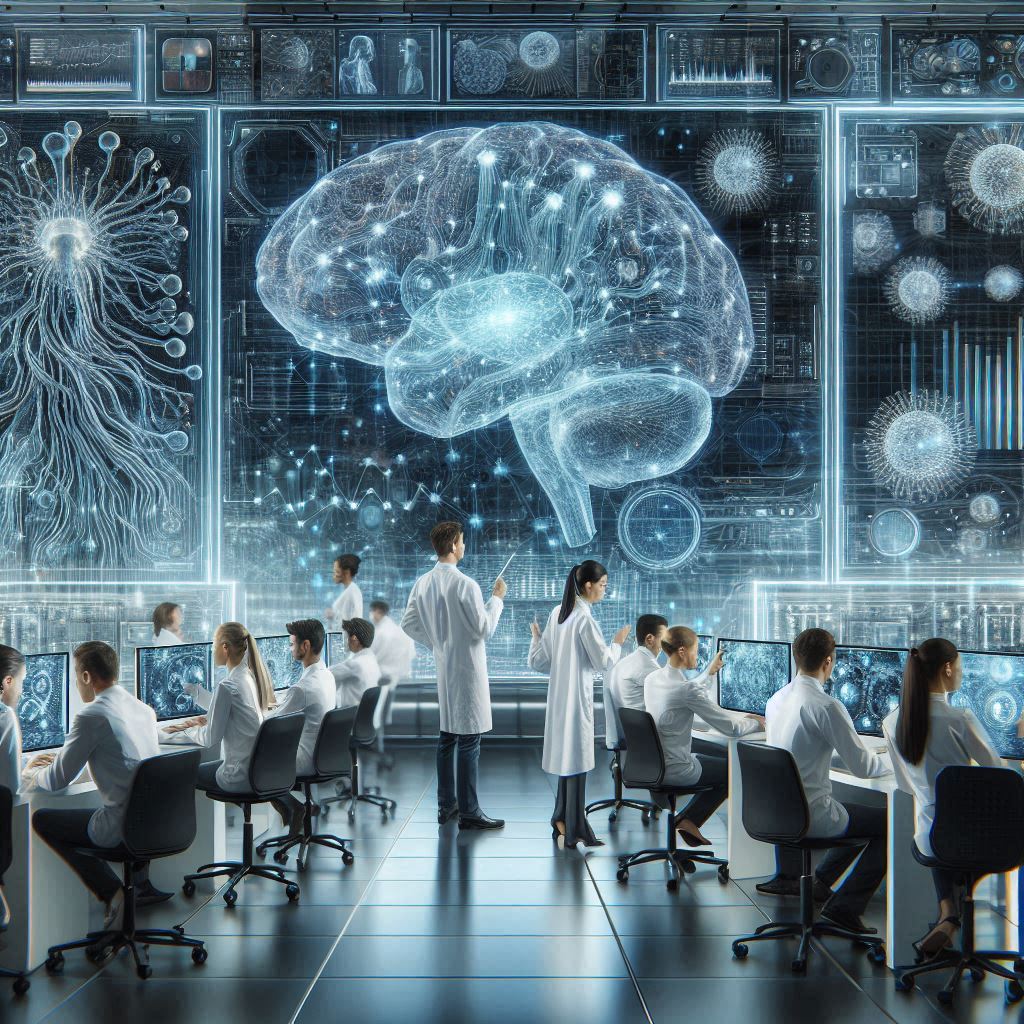
Core Components:
- Neurons: Simulated units that process and transmit information.
- Synapses: Connections between neurons that adjust their “strength” based on learning.
- Spikes: Electrical signals that carry information between neurons.
For example, when a neuromorphic – chip processes an image, it doesn’t analyze every pixel. Instead, it identifies patterns and features using event-driven spikes, much like how the human brain recognizes shapes and objects.
Advantages of Neuromorphic Computing
Neuromorphic-computing offers several unique advantages over traditional computing systems:
1. Energy Efficiency
Neuromorphic-chips consume significantly less power than conventional CPUs and GPUs, making them ideal for applications where energy consumption is a critical factor, such as edge computing and mobile devices.
2. Real-Time Learning
Unlike traditional AI systems, which require extensive training datasets and computational power, neuromorphic systems can learn and adapt in real-time, similar to the human brain.
3. Scalability
Neuromorphic architectures are inherently scalable, enabling the development of systems that can process massive amounts of data simultaneously.
4. Resilience
These systems are highly resilient to noise and disruptions, making them robust for real-world applications like autonomous vehicles and robotics.
Applications of Neuromorphic Computing
Neuromorphic-computing has the potential to revolutionize numerous industries and applications:
1. Healthcare
- Personalized Medicine: Neuromorphic systems can analyze complex biological data to develop personalized treatment plans.
- Brain-Machine Interfaces: These interfaces can help restore motor functions in patients with neurological disorders.
2. Artificial Intelligence
- Adaptive AI Systems: Neuromorphic AI can learn and adapt to new tasks without extensive retraining.
- Natural Language Processing (NLP): Enhanced NLP capabilities for real-time language translation and conversational AI.
3. Robotics
- Autonomous Robots: Neuromorphic-chips enable robots to process sensory data and make decisions in real-time.
- Human-Robot Interaction: Improved interaction between humans and robots through adaptive learning.
4. IoT and Edge Computing
- Smart Sensors: Neuromorphic chips can power energy-efficient sensors for smart cities and homes.
- Real-Time Analytics: Process data locally on edge devices, reducing latency and improving efficiency.
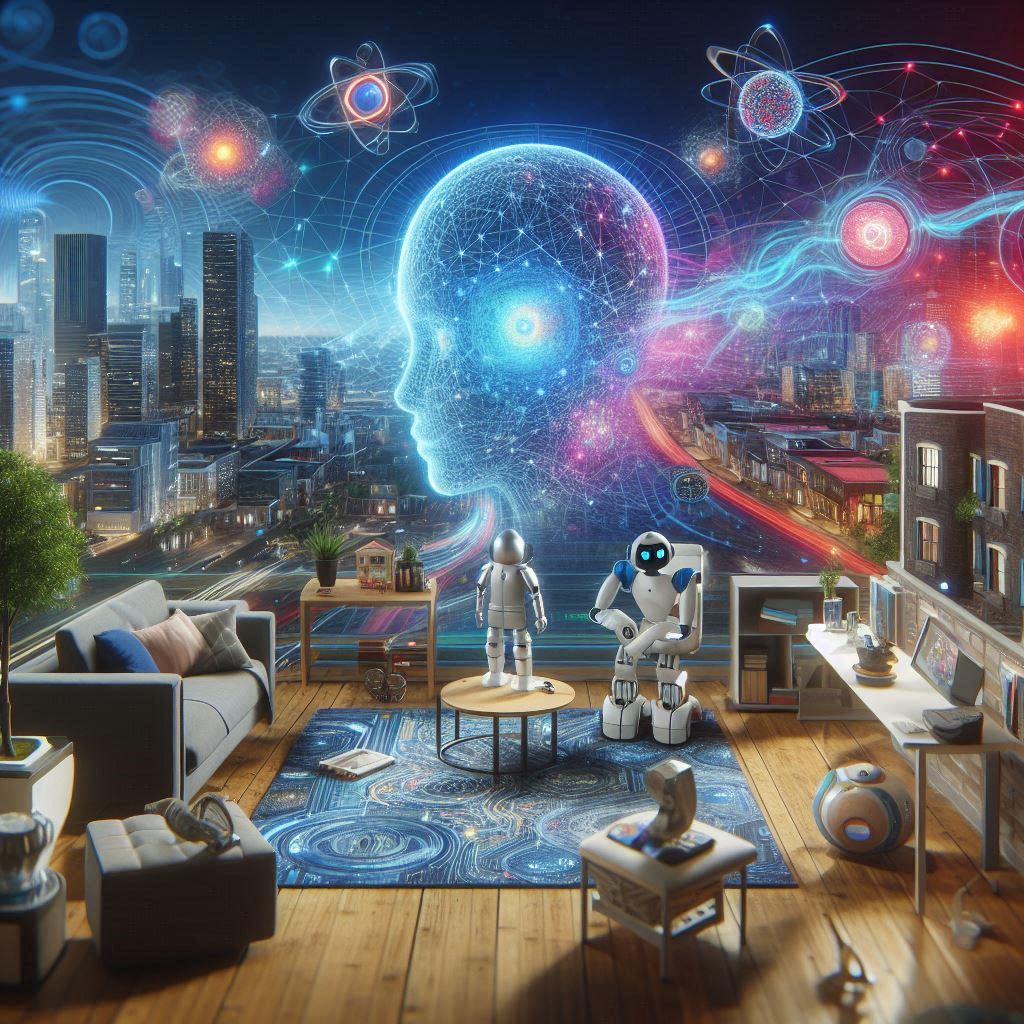
5. Cybersecurity
- Anomaly Detection: Neuromorphic systems can identify unusual patterns in network traffic, enhancing cybersecurity measures.
Challenges in Neuromorphic Computing
Despite its promise, neuromorphic-computing faces several challenges:
1. Hardware Limitations
Developing neuromorphic hardware that can effectively mimic the brain’s complexity is a significant challenge. Current technologies, such as memristors and phase-change materials, are still in the early stages of development.
2. Standardization
The lack of standardized frameworks and benchmarks makes it difficult to compare and evaluate different neuromorphic systems.
3. Scalability
While neuromorphic architectures are theoretically scalable, practical implementations often face issues related to heat dissipation and integration with existing systems.
4. Limited Ecosystem
The ecosystem for neuromorphic computing, including software tools and developer resources, is still nascent compared to traditional computing platforms.
Future Outlook
The future of neuromorphic computing is undeniably exciting. As research progresses, we can expect significant advancements in both hardware and software, enabling a wide range of new applications.
1. Collaboration with Neuroscience
Ongoing collaboration between computer scientists and neuroscientists will provide deeper insights into how the brain works, paving the way for more sophisticated neuromorphic systems.
2. Commercialization
Companies like Intel, IBM, and BrainChip are already making strides in neuromorphic computing, bringing us closer to practical, real-world applications.
3. Integration with AI
Neuromorphic_computing could play a pivotal role in advancing general AI, enabling machines to learn and adapt in ways that closely resemble human cognition.
Conclusion
Neuromorphic-computing represents a paradigm shift in the world of technology. By mimicking the brain’s structure and processes, it offers unparalleled efficiency, adaptability, and resilience. While challenges remain, the potential applications—from personalized medicine to autonomous robots—highlight the transformative power of this emerging field.
As we continue to push the boundaries of innovation, neuromorphic computing stands as a testament to what’s possible when technology takes inspiration from the wonders of nature. The journey has just begun, and the future is bound to be nothing short of extraordinary.